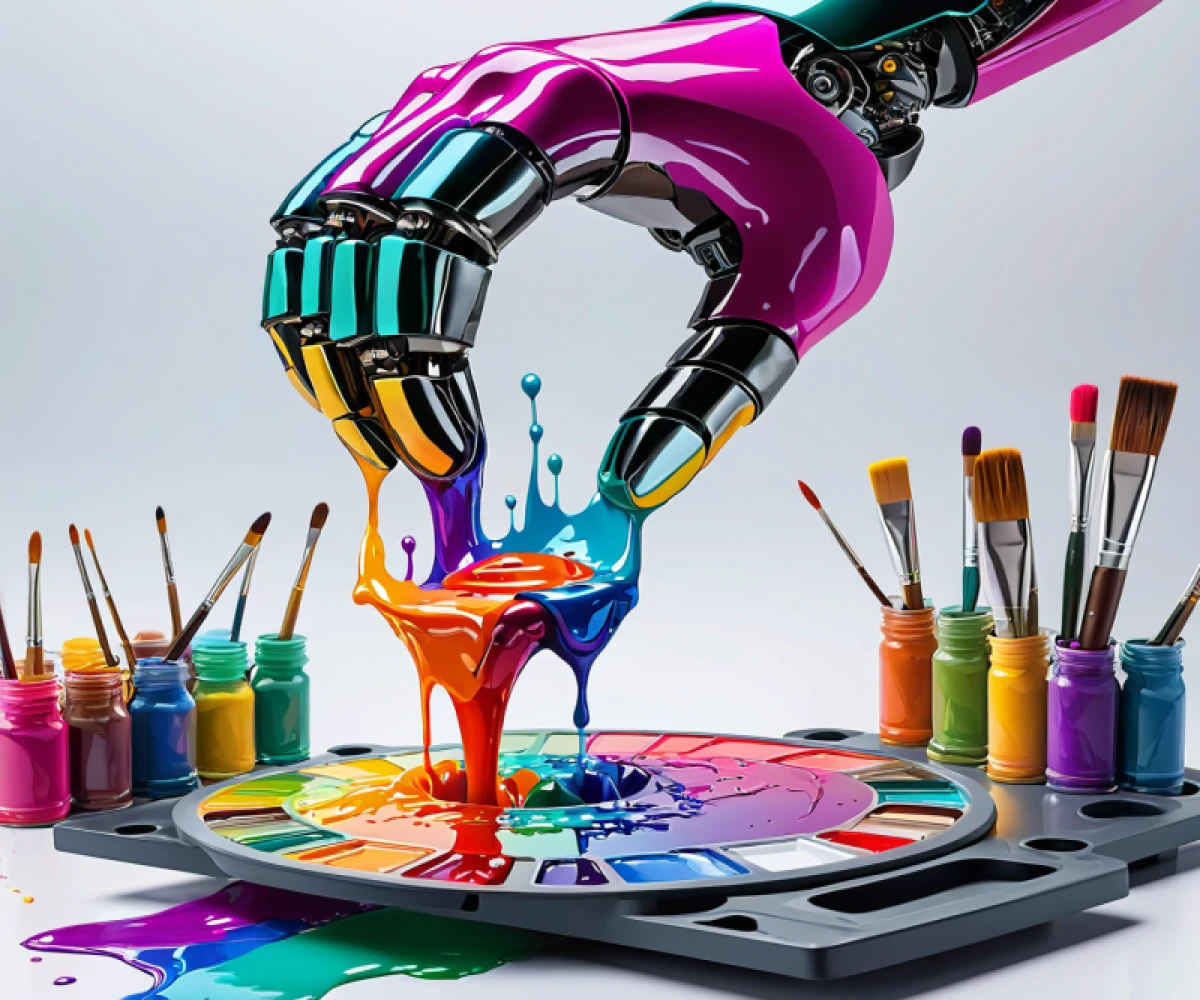
Generative AI vs. machine learning: How are they different?
Demystifying AI: Generative AI vs. Machine Learning - What's the Difference?
Artificial intelligence (AI) is a vast and exciting field, but within it lies a spectrum of specialized approaches. Two prominent players are generative AI and machine learning (ML), and while they might seem similar at first glance, their goals and functionalities differ significantly. Buckle up, as we delve into the key distinctions between these AI powerhouses!
The Core Mission:
- Machine Learning: Think of ML as the analyst and predictor. It ingests data, identifies patterns, and makes informed guesses about future events or classifications. Imagine a spam filter learning to recognize suspicious emails or a recommendation engine suggesting music you might enjoy.
- Generative AI: This creative force focuses on generating entirely new data, like text, images, or music. It analyzes existing content, understands its underlying patterns, and then uses that knowledge to produce novel creations. Think of generating realistic portraits, composing original songs, or writing unique blog posts.
Under the Hood:
- Learning Techniques: ML employs various strategies like supervised learning (learning from labeled data) or unsupervised learning (finding patterns without labels). Generative AI often uses techniques like Generative Adversarial Networks (GANs) where two models compete: one generating data, the other critiquing it, leading to constant refinement.
- Outputs: ML models spit out predictions, classifications, or inferences. Generative AI delivers brand new content, pushing the boundaries of what already exists.
- Performance Metrics: Evaluating ML success hinges on quantitative measures like accuracy, precision, and recall. Generative AI thrives on qualitative judgments like realism, coherence, and diversity.
Working Together:
While distinct, these AI subfields often collaborate. ML can prepare data for generative models, and generative outputs can be fed back into ML models for further learning. It's a dynamic partnership pushing the boundaries of AI capabilities.
So, where can we see them in action?
- Generative AI: Creating personalized marketing content, composing music for video games, generating realistic 3D models for architecture, and even writing creative fiction!
- Machine Learning: Facial recognition software, self-driving cars, medical diagnosis tools, and spam filters are just a few examples.
Remember: Generative AI and machine learning are both powerful tools, each with unique strengths and applications. Understanding their differences allows us to harness their potential and unlock the exciting possibilities they hold for our future.
Further Exploration:
- Want to dive deeper? Check out resources on GANs, VAEs, and other generative AI techniques.
- Explore the ethical considerations surrounding AI-generated content and potential biases.
- Stay curious and keep learning about the ever-evolving world of AI!
I hope this blog clarifies the distinctions between these fascinating AI subfields. Let me know if you have any further questions!